SNUx: Mathematical understanding of uncertainty
This lecture series discusses how the concept of probability can be used to handle, control, and exploit uncertainty in the real-world. It is an undergraduate-level lecture series on probability, but is entirely different from the usual courses on probability theory. The lectures cover the basics of probability theory including the relevant mathematics, but instead of focusing on mathematics, the lectures explain how probability theory can help understand real-world uncertainty using various examples. The examples are used to describe how uncertainty can be exploited to implement modern randomized algorithms such as Markov chain Monte Carlo and deep learning.
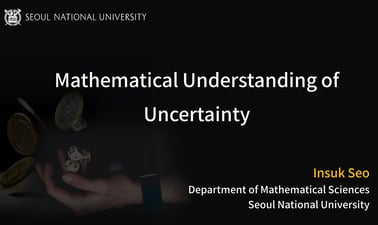
12 semanas
1–2 horas por semana
A tu ritmo
Avanza a tu ritmo
Este curso está archivado
Se anunciarán próximas fechas
Mathematical understanding of uncertainty
De un vistazo
- Institution SNUx
- Subject Matemáticas
- Level Introductory
- Prerequisites Ninguno
- Language English
- Video Transcript English
- Associated skillsCalculus, Probability, Algorithms, Markov Chain Monte Carlo, Basic Math, Deep Learning, Random Variables, Probability Theories, Markov Chain
¿Te interesa este curso para tu negocio o equipo?
Capacita a tus empleados en los temas más solicitados con edX para Negocios.